
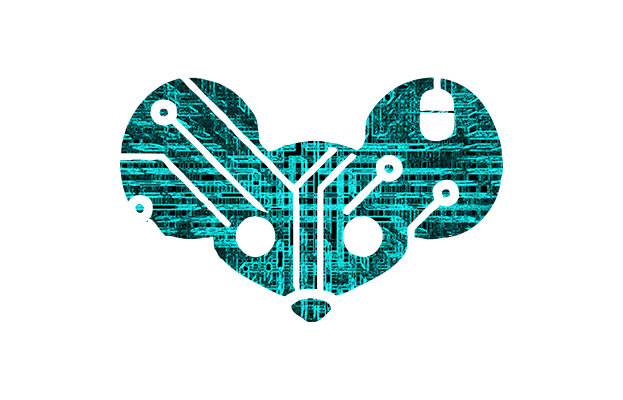
Lots of good comments here. I think there’s many reasons, but AI in general is being quite hated on. It’s sad to me - pre-GPT I literally researched how AI can be used to help people be more creative and support human workflows, but our pipelines around the AI are lacking right now. As for the hate, here’s a few perspectives:
- Training data is questionable/debatable ethics,
- Amateur programmers don’t build up the same “code muscle memory”,
- It’s being treated as a sole author (generate all of this code for me) instead of like a ping-pong pair programmer,
- The time saved writing code isn’t being used to review and test the code more carefully than it was before,
- The AI is being used for problem solving, where it’s not ideal, as opposed to code-from-spec where it’s much better,
- Non-Local AI is scraping your (often confidential) data,
- Environmental impact of the use of massive remote LLMs,
- Can be used (according to execs, anyways) to replace entry level developers,
- Devs can have too much faith in the output because they have weak code review skills compared to their code writing skills,
- New programmers can bypass their learning and get an unrealistic perspective of their understanding; this one is most egregious to me as a CS professor, where students and new programmers often think the final answer is what’s important and don’t see the skills they strengthen along the way to the answer.
I like coding with local LLMs and asking occasional questions to larger ones, but the code on larger code bases (with these small, local models) is often pretty non-sensical, but improves with the right approach. Provide it documented functions, examples of a strong and consistent code style, write your test cases in advance so you can verify the outputs, use it as an extension of IDE capabilities (like generating repetitive lines) rather than replacing your problem solving.
I think there is a lot of reasons to hate on it, but I think it’s because the reasons to use it effectively are still being figured out.
Some of my academic colleagues still hate IDEs because tab completion, fast compilers, in-line documentation, and automated code linting (to them) means you don’t really need to know anything or follow any good practices, your editor will do it all for you, so you should just use vim or notepad. It’ll take time to adopt and adapt.
Insane compute wasn’t everything. Hinton helped develop the technique which allowed more data to be processed in more layers of a network without totally losing coherence. It was more of a toy before then because it capped out at how much data could be used, how many layers of a network could be trained, and I believe even that GPUs could be used efficiently for ANNs, but I could be wrong on that one.
Either way, after Hinton’s research in ~2010-2012, problems that seemed extremely difficult to solve (e.g., classifying images and identifying objects in images) became borderline trivial and in under a decade ANNs went from being almost fringe technology that many researches saw as being a toy and useful for a few problems to basically dominating all AI research and CS funding. In almost no time, every university suddenly needed machine learning specialists on payroll, and now at about 10 years later, every year we are pumping out papers and tech that seemed many decades away… Every year… In a very broad range of problems.
The 580 and CUDA made a big impact, but Hinton’s work was absolutely pivotal in being able to utilize that and to even make ANNs seem feasible at all, and it was an overnight thing. Research very rarely explodes this fast.
Edit: I guess also worth clarifying, Hinton was also one of the few researching these techniques in the 80s and has continued being a force in the field, so these big leaps are the culmination of a lot of old, but also very recent work.